Ph.D. Theses
The doctoral dissertation represents the culmination
of the entire graduate school experience. It is a snapshot of all that
a student has accomplished and learned about their dissertation topics.
While we could post these on our publications page, we feel that they
deserve a page of their own. Here are Ph.D. theses from lab members
in reverse chronological order.
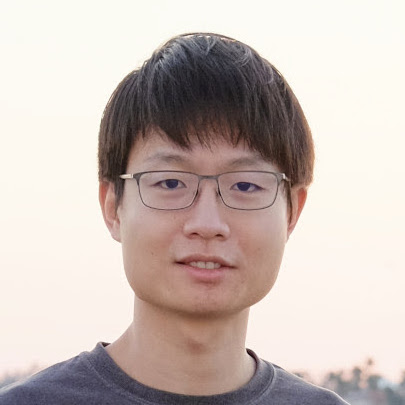 |
Deep Neural Networks for 3D Processing and High-Dimensional Filtering by Hang Su, May 2020.
[pdf]
|
Abstract:
Deep neural networks (DNN) have seen tremendous success in the past few years, advancing state of the art in many AI areas by significant margins. Part of the success can be attributed to the wide adoption of convolutional filters. These filters can effectively capture the invariance in data, leading to faster training and more compact representations, and at the same can leverage efficient parallel implementations on modern hardware. Since convolution operates on regularly structured grids, it is a particularly good fit for texts and images where there are inherent rigid 1D or 2D structures. However, extending DNNs to 3D or higher-dimensional spaces is non-trivial, because data in such spaces often lack regular structure, and the curse of dimensionality can also adversely impact performance in multiple ways.
In this thesis, we present several new types of neural network operations and architectures for data in 3D and higher-dimensional spaces and demonstrate how we can mitigate these issues while retaining the favorable properties of 2D convolutions. First, we investigate view-based representations for 3D shape recognition. We show that a collection of 2D views can be highly informative, and we can adapt standard 2D DNNs with a simple pooling strategy to recognize objects based on their appearances from multiple viewing angles with unprecedented accuracies. Our next study makes a connection between 3D point cloud processing and sparse high-dimensional filtering. The resulting representation is highly efficient and flexible, and enables native 3D operations as well as joint 2D-3D reasoning. Finally, we show that high-dimensional filtering is also a powerful tool for content-adaptive image filtering. We demonstrate its utility in computer vision applications where preserving sharp details in output is critical, including joint upsampling and semantic segmentation.
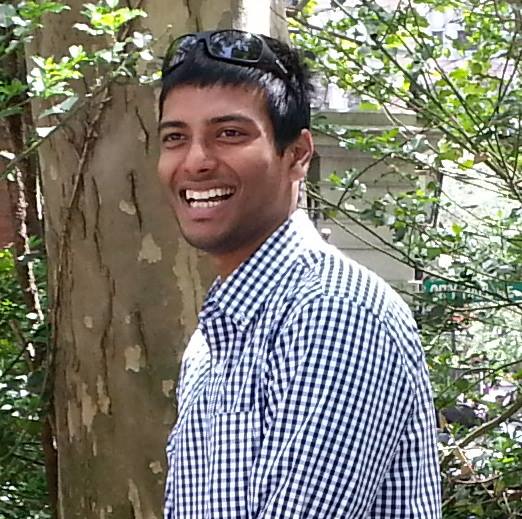 |
Improving Visual Recognition with Unlabeled Data by Aruni RoyChowdhury, May 2020.
[pdf]
|
Abstract:
The success of deep neural networks has resulted in computer vision systems that obtain high accuracy on a wide variety of tasks such as image classification, object detection, semantic segmentation, etc.
However, most state-of-the-art vision systems are dependent upon large amounts of labeled training data, which is not a scalable solution in the long run.
This work focuses on improving existing models for visual object recognition and detection without being dependent on such large-scale human-annotated data.
We first show how large numbers of hard examples (cases where an existing model makes a mistake) can be obtained automatically from unlabeled video sequences by exploiting temporal consistency cues in the output of a pre-trained object detector.
These examples can strongly influence a model's parameters when the network is re-trained to correct them, resulting in improved performance on several object detection tasks.
Further, such hard examples from unlabeled videos can be used to address the problem of unsupervised domain adaptation. We focus on the automatic adaptation of an existing object detector to a new domain with no labeled data, assuming that a large number of unlabeled videos are readily available.
Finally, we address the problem of face recognition, which has achieved high accuracy by employing deep neural networks trained on massive labeled datasets. Further improvements through supervised learning require significantly larger datasets and hence massive annotation efforts. We improve upon the performance of face recognition models trained on large-scale labeled datasets by using unlabeled faces as additional training data. We present insights and recipes for training deep face recognition models with labeled and unlabeled data at scale, addressing real-world challenges such as overlapping identities between the labeled and unlabeled datasets, as well as label noise introduced by clustering errors.
 |
Motion Segmentation - Segmentation of Independently Moving Objects in Video by Pia Katalin Bideau, Feburary 2020.
[pdf]
|
Abstract:
The ability to recognize motion is one of the most important functions of our visual system.
Motion allows us both to recognize objects and to get a better understanding of the 3D world in which we are moving. Because of its importance, motion is used to answer a wide variety of fundamental questions in computer vision such as: (1) Which objects are moving independently in the world? (2) Which objects are close and which objects are far away? (3) How is the camera moving?
My work addresses the problem of moving object segmentation in unconstrained videos. I developed a probabilistic approach to segment independently moving objects
[ArXiv] in a video sequence, connecting aspects of camera motion estimation, relative depth and flow statistics.
My work consists of three major parts:
- Modeling motion using a simple (rigid) motion model strictly following the principles of perspective projection and segmenting the video into its different motion components by assigning each pixel to its most likely motion model in a Bayesian fashion. [ECCV16]
- Combining piecewise rigid motions to more complex, deformable and articulated objects, guided by learned semantic object segmentations. [CVPR18]
- Learning highly variable motion patterns using a neural network trained on synthetic (unlimited) training data. Training data is automatically generated strictly following the principles of perspective projection. In this way well-known geometric constraints are precisely characterized during training to learn the principles of motion segmentation rather than identifying well-known structures that are likely to move. [ECCV18 workshop]
This work shows that a careful analysis of the motion field not only leads to a consistent segmentation of moving objects in a video sequence, but also helps us understand the scene geometry of the world we are moving in.
 |
Higher-Order Representations for Visual Recognition by Tsung-Yu Lin, Feburuary 2020.
[pdf]
|
Abstract:
In this thesis, we present a simple and effective architecture called Bilinear Convolutional Neural Networks (B-CNNs). These networks represent an image as a pooled outer product of features derived from two CNNs and capture localized feature interactions in a translationally invariant manner. B-CNNs generalize classical orderless texture-based image models such as bag-of-visual-words and Fisher vector representations. However, unlike prior work, they can be trained in an end-to-end manner. In the experiments, we demonstrate that these representations generalize well to novel domains by fine-tuning and achieve excellent results on fine-grained, texture and scene recognition tasks. The visualization of fine-tuned convolutional filters shows that the models are able to capture highly localized attributes. We present a texture synthesis framework that allows us to visualize the pre-images of fine-grained categories and the invariances that are captured by these models.
In order to enhance the discriminative power of the B-CNN representations, we investigate normalization techniques for rescaling the importance of individual features during aggregation. Spectral normalization scales the spectrum of the covariance matrix obtained after bilinear pooling and offers a significant improvement. However, the computation involves singular value decomposition, which is not computationally efficient on modern GPUs. We present an iteration-based approximation of matrix square-root along with its gradients to speed up the computation and study its effect on fine-tuning deep neural networks. Another approach is democratic aggregation, which aims to equalize the contributions of individual feature vector into the final pooled image descriptor. This achieves a comparable improvement, and can be approximated in a low-dimensional embedding unlike the spectral normalization. Therefore, this approach is friendly to aggregating higher-dimensional features. We demonstrate that the two approaches are closely related, and we discuss their trade-off between performance and efficiency.
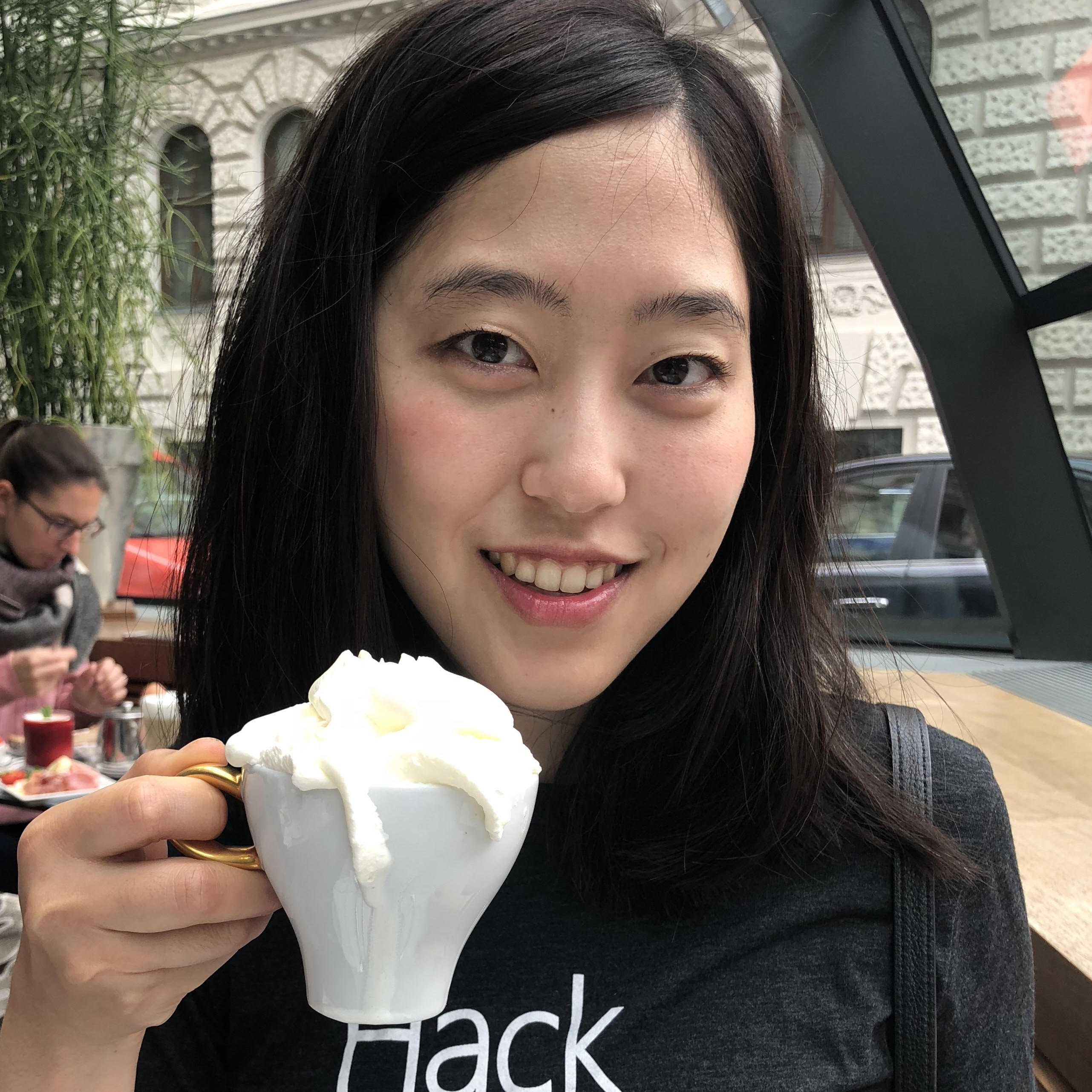 |
Improving Face Clustering in Videos by SouYoung Jin, Feburuary 2020.
[pdf]
|